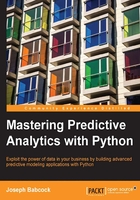
Chapter 2. Exploratory Data Analysis and Visualization in Python
Analytic pipelines are not built from raw data in a single step. Rather, development is an iterative process that involves understanding the data in greater detail and systematically refining both model and inputs to solve a problem. A key part of this cycle is interactive data analysis and visualization, which can provide initial ideas for features in our predictive modeling or clues as to why an application is not behaving as expected.
Spreadsheet programs are one kind of interactive tool for this sort of exploration: they allow the user to import tabular information, pivot and summarize data, and generate charts. However, what if the data in question is too large for such a spreadsheet application? What if the data is not tabular, or is not displayed effectively as a line or bar chart? In the former case, we could simply obtain a more powerful computer, but the latter is more problematic. Simply put, many traditional data visualization tools are not well suited to complex data types such as text or images. Additionally, spreadsheet programs often assume data is in a finalized form, whereas in practice we will often need to clean up the raw data before analysis. We might also want to calculate more complex statistics than simple averages or sums. Finally, using the same programming tools to clean up and visualize our data as well as generate the model itself and test its performance allows a more streamlined development process.
In this chapter we introduce interactive Python (IPython) notebook applications (Pérez, Fernando, and Brian E. Granger. IPython: a system for interactive scientific computing. Computing in Science & Engineering 9.3 (2007): 21-29). The notebooks form a data preparation, exploration, and modeling environment that runs inside a web browser. The commands typed in the input cells of an IPython notebook are translated and executed as they are received: this kind of interactive programming is helpful for data exploration, where we may refine our efforts and successively develop more detailed analyses. Recording our work in these Notebooks will help to both backtrack during debugging and serve as a record of insights that can be easily shared with colleagues.
In this chapter we will discuss the following topics:
- Reading raw data into an IPython notebook, cleaning it, and manipulating it using the Pandas library.
- Using IPython to process numerical, categorical, geospatial, or time-series data, and perform basic statistical analyses.
- Basic exploratory analyses: summary statistics (mean, variance, median), distributions (histogram and kernel density), and auto-correlation (time-series).
- An introduction to distributed data processing with Spark RDDs and DataFrames.